All published articles of this journal are available on ScienceDirect.
A Binary Mixture of Sesame And Castor Oil as an Ecofriendly Corrosion Inhibitor of Mild Steel In Crude Oil
Abstract
Background:
A binary mixture of sesame and castor oil was used for reducing the corrosion rate of mild steel in crude oil environments. This study investigated the corrosion behavior of a binary mixture of sesame and castor oil as a corrosion inhibitor for mild steel in crude oil. Different parameters such as immersion time, the concentration of inhibitor and pH were investigated for corrosion of mild steel.
Methods:
Experimental analysis indicates that a passive layer of the inhibitor formed over the surface of mild steel thereby reducing the corrosion rate. This was demonstrated by varying different process parameters such as the concentration of binary inhibitor, pH and time using two different statistical models; the Response Surface Methodology (RSM) and the Artificial Neural Network (ANN).
Results:
From the results, it was observed that ANN was a better predictive tool to determine the corrosion rate of mild steel than the RSM.
Conclusion:
Overall, both the models prove that relative to the process parameters used, the importance level of the parameters was Time < Concentration of binary inhibitor < pH.
1. INTRODUCTION
Investigation of corrosion prevention techniques in oilfield applications is essential due to the occurrence of persistent corrosion problems, which largely contribute to huge production expenses of oil and gas exploration around the world. However, suitable corrosion control measures can help avert several potential disasters that can result in crucial issues, including environmental pollution, water resource, negative social impacts, and loss of life. Corrosion exists in oilfields at all stages, including surface to downhole and in processing facilities. It occurs as perforations in tanks, pipelines, tubing, casing as well as other equipment [1, 2]. Corrosion problems are typically linked to equipment maintenance and operating problems resulting in frequent partial as well as the total shutdown of the process, leading to critical economic losses [3]. Hydrocarbons such as natural gas condensates and crude oil are weak electrolytes, thus have high resistivity. Oilfield corrosion is mostly induced by the presence of chemicals in the water phase lowering the inherent water resistivity [4]. Various techniques have been used to reduce the rate at which corrosion occurs. The application of material resisting corrosion, coatings, cathodic protection and incorporation of corrosion inhibitors are the four methods practically applied for corrosion control. The use of inhibitors has been generally proven as the most efficient, economical and practical method in preserving oil wells against corrosion [5].
Inhibitors have been primarily utilized as an atypical treatment for corrosion owing to previous studies that have reported its successful use and cost-effectiveness [6, 7]. Inhibitors can operate as an active component by forming a protective covering on the surface of a substrate, altering their surface properties [8]. The existence of crude oil has been reported to reduce the effectiveness of an inhibitor in reducing the corrosion of metals such as carbon steel in contrast to corrosion in the absence of crude oil [4]. Accumulation of corrosion inhibitors in emulsion droplets of the oil/water interface can remarkably reduce the concentration of the inhibitor in the water phase. Presumably, it has been estimated that inhibitors can be exhausted from the water phase if the surface area of the total oil droplets surpasses 1 m2/liter (magnitude). Crucial emulsification of inhibitor results in a higher risk of corrosion and exorbitant inhibitor consumption. The inhibitor sensitivity in oil-in-water emulsions is reliant on the type of inhibitor used [9]. Under specific situations, oil-in-water emulsions may encourage oil wetting, thereby resulting in better protection against corrosion [9].
Mild steel is a frequently used material and is extensively used in numerous industries. The steel used in the construction of oil wells includes API N80 mild steel (API – American Petroleum Institute), L80 and J55, to high Cr-content residual alloys, like austenitic –ferric steel, for instance, modified martensitic 13% Cr steel, referred to as Super-13 [3]. As a result of the cost, availability, and high mechanical strength, mild steel is frequently used in oilfield industries for the construction of pipes, storage tanks and processing equipment [10]. A major drawback of mild steel application is that it undergoes corrosion when subjected to several operating environments [11]. Liquids produced in oilfields are usually transmitted by different metals in direct contact with each other. Surface pump assemblies and downhole usually combine stainless steel, low-alloy steels, carbon steels, steels plated by other metals, copper and aluminum alloys. Occasionally, these galvanic couples result in corrosion problems [12]. Corrosion of mild steel substrate entails various reduction and oxidation reactions and adequate O2 and H2O are vital for corrosion of the metallic substrate. Organic inhibitors act by providing a coating on the surface of the metal; therefore, for coated substrates, corrosion occurs through these three steps: (1) adsorption of water on coating which can be controlled through surface hydrophobicity; (2) water diffusion through coating which occurs as a consequence of accumulation of defects in the coating; and (3) corrosive species and reaching water at the interface of the coating/metal influenced by the strength of the bond of the coating on the metallic substrate [13]. Several types of inhibitors have been used on mild steel to inhibit the rate at which corrosion occurs [3, 10, 14].
This study aims to investigate the corrosion of mild steel in crude oil, in the presence of a binary mixture of sesame and castor oil as a corrosion inhibitor. Systematic studies on the effect of a binary mixture of sesame and castor oil have been reported by Oyekunle [15] in brine environments but this study considers the effect of the binary mixture in crude oil environments. The main objective of this study is to determine the inhibitory effect of this binary mixture (sesame and castor oil) on mild steel in crude oil, to investigate the effect of process variables (concentration of binary mixture, pH of the crude oil, and time) and the response (corrosion rate); and to obtain optimum conditions for minimum corrosion rate of mild steel in crude oil using binary mixture of sesame and castor oil. Thus, Box-Behnken Design (BBD) - response surface methodology (RSM) was utilized in this study. The resulting experimental data was analyzed by Neural version 2.5 (CPX-Software). The results obtained from both the models (ANN and RSM) were compared for better predictability of the corrosion rate responses considering their limitations and implementations. The advantage of this method is the concurrent evaluation of interactive and individual effects of process variables on the response based on a limited number of experiments [16, 17].
2. EXPERIMENTAL
2.1. Test Materials
Mild steel composition of C = 0.22; Si = 0.30; Mn = 0.65; Cu = 0.030; P = 0.045 and Fe balance. Mild steel sheet thickness of 3.0 mm was cut into coupons of 20 × 20 mm to carry out several tests. The mild steel surfaces were mechanically abraded with 400 grade of emery papers before it was used for the immersion tests. Before the immersion corrosion tests, the specimens were cleaned in acetone and purified water.
2.2. Test Solutions
Crude oil used was brought from an oil field in Rivers state, Nigeria. The pH of the crude oil environment was adjusted using 0.1 mol / L HCl and 0.1 mol/ L NaCl solutions. Before the immersion experiments, the pH of the crude oil solution was measured by pH meter.
2.3. Immersion Tests And Design of Experiments
Immersion test experiments were performed using Minitab 17 statistical software to vary the process input variables into different levels to observe their effect on the corrosion rate. The result obtained from the experimental design matrix was evaluated using Box Behnken Design to derive mathematical model by applying RSM quadratic model and the same data obtained from Box Behnken Design was employed to train ANN utilizing normal multilayer feed-forward neural network.
In this study, mild steel coupons were immersed in crude oil at 27 °C, and the effect of pH,concentration of the binary inhibitor, and the time of the corrosion rate were investigated. Immersion tests were performed with three replicates of each experimental run designed as presented in Table 1. The weight of steel coupons before and after the tests was estimated using an electronic balance (Adventurer Ohaus SNR: 8728406387) to observe weight change. Corrosion rates were calculated based on the loss in the weight of the mild steel by Eq. (1) in accordance with the ASTM G31 standard [18, 19].
![]() |
(1) |
Where WL = weight loss (g), A = area of the mild steel specimen (cm2), t = time (year(y)), and ρ = density (g/ cm3).
The experimental design was based on three factors and three levels as illustrated in Table 1. The table presents actual and coded variables, and 15 experimental runs were defined by Box Behnken design (BBD). The quality of the model fitness was determined by a test of significance and analysis of variance (ANOVA). The equation for the second-order polynomial was obtained from the interactional relationship of variables. The quadratic response model was fitted as given in Eq. (2).
![]() |
(2) |
Where Y is the response predicted value (corrosion rate), where d0 represents offset term, d1, d2, and d3 represent the linear coefficients, d11, d22, and d33 are quadratic terms while d12, d13, and d23 are cross products. The experiments were conducted in triplicates and the average value was used. The experimental data of BBD design matrix and their experimental responses are tabulated in Table 2. Minitab 17 statistical software was utilized for designing experimental sets and analysis of data using response surface methodology-Box Behnken design.
2.4. Artificial Neural Networks (ANN)
In this training procedure, a multilayer normal feed-forward network was applied to process information from the input layer through the hidden layer to the output layer. The output of the designated layer was obtained from the output layer [17]. The multilayer normal feed-forward network was used to determine the inhibitive property of the binary mixture of sesame and castor oil on the corrosion rate of mild steel in a crude oil environment. This statistical approach uses superintend learning known as backpropagation throughout the training period of the model. Training of the learning works as a result of the changes observed in the connection weights, as established by the errors calculated from the observed values, proceeding from the output and advancing to the input. Feedforward propagation was applied by the ANN, in this case, each layer progresses to the next layer without regress (Fig. 1). ANN input values are classified into categorical values as pH, the concentration of binary inhibitor and time, while the corrosion rate is the ANN output.
ANN performance was assessed by the error and accurate values of the model. The root mean square error (RMSE) and the coefficient of determination (R2) were estimated by Eqs. (3 and 4) respectively [20].
![]() |
(3) |
![]() |
(4) |
Where n represents the number of observations, xi, represents the experimental corrosion rate (mm/ yr), and yi represents the corrosion rate estimated by the model. These criteria were applied to determine the performance of ANN in modelling of the corrosion rate of mild steel in crude oil environments using sesame and castor oil as a binary inhibitor due to the quantitative variables presented by the output and input data, since these criteria are frequently used in evaluating the model's performance. The weights and bias for the output and hidden layer used in this study are illustrated in Table 3.
3. RESULTS AND DISCUSSION
3.1. Corrosion Inhibition of Mild Steel
The results of the corrosion rate of identical mild steel coupons in different concentrations of binary inhibitor, time and pH of the crude oil solution are reported in Table 2. Table 2 reveals that the highest corrosion rate of mild steel (00.98 mm/yr) was observed at a low pH of 5, and binary inhibitor concentration of 23 mg/L over a period of 21 days. This can be as a result of the length of time which was 21 days; the layer provided by the inhibitor would have degraded at a fast rate. While the lowest corrosion rate of 0.04 mm/yr was observed at the maximum concentration of binary inhibitor used in this study which was 30 mg/L and a shorter length of time of 13 days, occurring at the same pH when the maximum corrosion rate was reported. This illustrates that the higher concentration of binary inhibitor and the lower reaction time at a low pH of 5 favor the reduction of the corrosion rate on mild steel in crude oil solutions.
Variables | Coded Symbol | Range and Levels | ||||
-2 | -1 | 0 | 1 | 2 | ||
pH | X | 3 | 5 | 6 | 7 | 8 |
Concentration of Binary Inhibitor (mg/ L) | Y | 15 | 19 | 23 | 26 | 30 |
Time (Days) | Z | 3 | 8 | 13 | 17 | 21 |
Sample No. | pH | The concentration of the Binary Inhibitor (mg /L) | Time (Days) | Experimental Corrosion Rate (mm /yr) | Predicted Values | Residual Values | ||
RSM | ANN | RSM | ANN | |||||
1 | 8 | 23 | 13 | 0.32 | 0.33 | 0.33 | -0.01 | 0.01 |
2 | 5 | 23 | 3 | 0.13 | 0.33 | 0.12 | -0.20 | 0.01 |
3 | 5 | 15 | 13 | 0.58 | 0.81 | 0.58 | -0.23 | 0.00 |
4 | 6 | 19 | 17 | 0.85 | 0.74 | 0.85 | 0.11 | 0.00 |
5 | 5 | 23 | 21 | 0.98 | 0.78 | 0.99 | 0.20 | 0.01 |
6 | 6 | 26 | 17 | 0.09 | 0.33 | 0.08 | -0.24 | 0.01 |
7 | 6 | 26 | 8 | 0.05 | 0.07 | 0.07 | -0.02 | 0.02 |
8 | 4 | 26 | 17 | 0.08 | 0.33 | 0.09 | -0.25 | 0.01 |
9 | 4 | 19 | 8 | 0.69 | 0.49 | 0.70 | 0.20 | 0.01 |
10 | 7 | 23 | 13 | 0.24 | 0.33 | 0.23 | -0.09 | 0.01 |
11 | 4 | 26 | 8 | 0.29 | 0.07 | 0.28 | 0.22 | 0.01 |
12 | 6 | 19 | 8 | 0.58 | 0.49 | 0.58 | 0.09 | 0.00 |
13 | 4 | 19 | 17 | 0.81 | 0.74 | 0.81 | 0.07 | 0.00 |
14 | 3 | 23 | 13 | 0.37 | 0.33 | 0.36 | 0.04 | 0.01 |
15 | 5 | 30 | 13 | 0.04 | -0.09 | 0.04 | 0.13 | 0.00 |
Layer | Weights of the Hidden Layer | Output (Corrosion rate mm/yr) | ||
1 | 2 | 3 | ||
Neuron 1 | 0.0142 | -1.2678 | -0.3241 | 2.2032 |
Neuron 2 | -4.0395 | -2.9931 | -0.5543 | 1.8634 |
Neuron 3 | 8.7337 | 2.1106 | 3.3179 | -2.0652 |
Bias | 4.1762 | -3.291 | 1.9324 | 0.7012 |
3.2. Molding Using Box Behnken Design – RSM & ANN
According to experimental results, equation 5 shows an empirical relationship between the response (i.e. experimental corrosion rate) and independent variables (Concentration of binary inhibitor, Time and pH). In the equation, the pH is not represented by the quadratic model, this further validates the less effect of the pH in crude oil solutions.
![]() |
(5) |
Where X represents the concentration of binary inhibitor, and Y represents time.
The predicted values of the response (experimental corrosion rate) have been calculated by the quadratic model from Eq. (5) and are presented in Table 2. Table 2 shows a clear agreement between the RSM predicted values, ANN predicted and the experimental values; this thereby demonstrates the reliability of both the models in predicting the corrosion rate of mild steel in crude oil solutions. The ANOVA results were summarized for the quadratic model indicating the adequacy and significance of the model given in Table 4. The large F – value and low P-value of the model imply that the model is significant [15, 17, 21]. The determination coefficient (R2) and the adjusted coefficient of determination (R2) were 72. 95% and 65.57% respectively, thereby indicating a reasonable fitness of the model to the experimental data. R2 of 72.95% implies that 72.95% of the variations experienced could adequately be illustrated by the fitted model in Eq. (5). The optimum corrosion rate of 0.04 mm/yr observed was at pH 5, at 30 mg/L concentration of the binary inhibitor over a period of 13 days. This validates the efficiency of the binary inhibitor in reducing the corroding potential of mild steel in crude oil solutions.
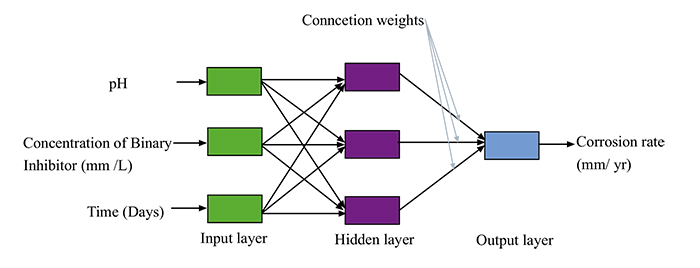
3.3. Effect Of Concentration of Binary Inhibitor And Time
The effect of the binary inhibitor concentration and time on the experimental corrosion rate is displayed in Fig. (2) From the figure, it can be observed that at constant pH, the corrosion rate increased as time increased. For instance, with a constant binary inhibitor of 23 mg/L, at a pH of 5, the corrosion rate increased from 0.13 to 0.98 mm/yr when the time of exposure increased from 3 to 17 days. Also, increasing the concentration of the binary mixture at constant pH and time also affected the corrosion rate i.e. corrosion rate of mild steel reduces from 0.85 to 0.09 mm/yr, when the concentration of the binary mixture increased from 19 to 26 at a constant pH and time period of 17 days. This shows the effect of a binary mixture; the binary composition of sesame and castor oil forms a passive layer over the surface of the mild steel inhibiting the corrosion rate. The more the concentration of the binary mixture, the greater the thickness of the passive layer formed over the surface of the mild steel. Also, since the higher reaction time was also responsible for the rapid degradation of the mild steel surface, therefore, it can be said that a higher concentration of the binary inhibitor requires a longer period of time for the passive layer to be completely degraded. Consequently, in order to prevent the rapid degradation of mild steel in the crude oil environment, greater attention is required when considering the amount of the inhibitor to be used and the length of time the equipment is meant to serve.
3.4. Effect Of pH And Concentration of Binary Inhibitor
Fig. (3) illustrates the effects of pH and the concentration of the binary inhibitor on mild steel at a constant time. The figure shows a decrease in the corrosion rate when the concentration of binary inhibitor increased. For instance, the corrosion rate of mild steel decreased from 0.58 to 0.04 mm/yr when the concentration of the binary inhibitor doubled at a constant pH of 5. Also at a constant pH of 4, the corrosion rate greatly reduced from 0.81 to 0.08 when the concentration of the binary inhibitor increased from 19 to 26 mm/yr. This implies that at an acidic concentration of the crude oil, the presence of a higher concentration of binary inhibitor was required to reduce the corrosion rate of mild steel, thus increasing its shelf life. This was in concordance with what was required at the near neutral pH of 6, whereby the corrosion rate reduced from 0.58 to 0.05 mm/yr when the concentration of the binary mixture increased from 19 to 26 mg/L. This also confirms that a broader passive layer of the inhibitor is formed over the surface of the mild steel at a higher concentration of brine, thus inhibiting the effect of crude oil as a corrosive agent on the mild steel.



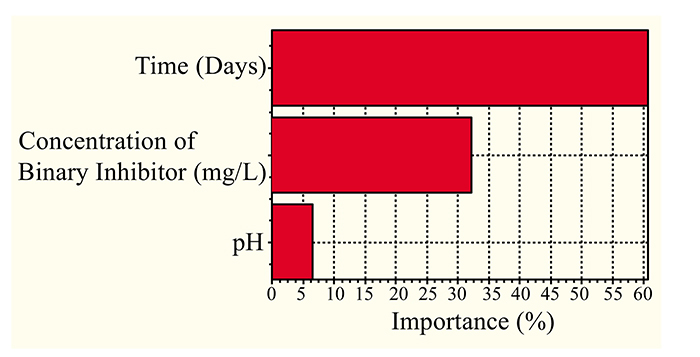
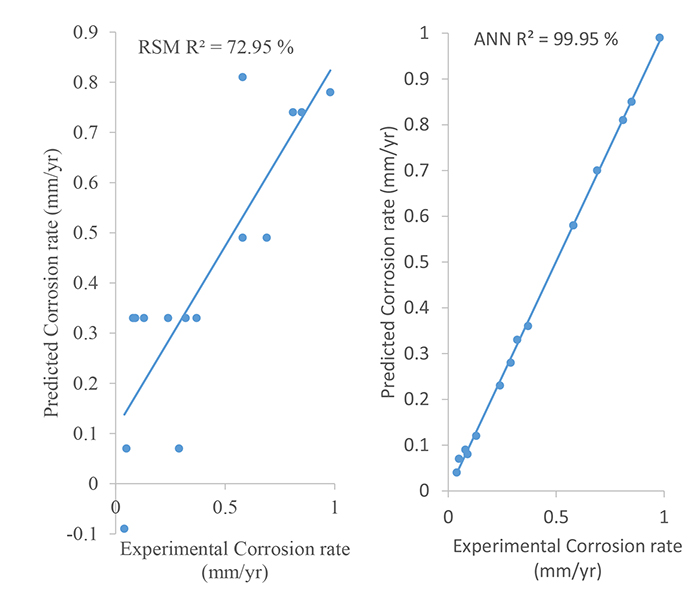
Source | Sum of Squares | DF | Contribution (%) | Adj. SS | Adj. MS | F-value | P-value |
Model | 1.03863 | 3 | 72.95 | 1.03863 | 0.34621 | 9.89 | 0.002 |
X | 0.74133 | 1 | 52.07 | 0.75103 | 0.75103 | 21.45 | 0.001 |
Y | 0.20904 | 1 | 14.68 | 0.20109 | 0.20109 | 5.74 | 0.035 |
Y2 | 0.08826 | 1 | 6.20 | 0.08826 | 0.08826 | 2.52 | 0.141 |
Error | 0.38511 | 11 | 27.05 | 0.38511 | 0.03501 | - | - |
Total | 1.42373 | 14 | 100.00 | - | - | - | - |
3.5. Effect Of Time And pH On The Corrosion Rate
The effect of crude oil pH and mild steel exposure time on crude oil environments was considered at a constant concentration of binary inhibitor in Fig. (4). Fig. (4) illustrates that at a constant concentration of the binary mixture and time, the corrosion rate decreased from 0.37 to 0.24 mm/yr when the pH increased from 3 to 7. Increasing the pH further to 8, the corrosion rate increased to 0.32 mm/yr. This shows that the pH has a very minimal effect on the corrosion rate; this was further explained in Fig. (5), which demonstrates a low significance level of 6.88% when compared to significance level of time (60.64%) and concentration of binary inhibitor (32.48%). At a constant pH of 5, the corrosion rate increased from 0.13 to 0.98 mm/L when the time increased from 3 to 21 days. This illustrates that at a longer reaction time, the passive layer formed by the binary inhibitor depletes. Moreover, the continuous exposure of mild steel surface to the corrosive environment gradually eliminates the presence of any corrosive layer formed on the mild steel by the binary inhibitor. This implies that in order to prevent future problems, the binary inhibitor should be reapplied into the corrosive environments.
3.6. Comparison of ANN And RSM Models
The determination coefficient (R2) by ANN was observed to be 99.95%; this was far greater than what was observed by the RSM model. This implies that a greater variation of 99.95% can be explained by the ANN model while a lesser variation of 72.95% can be explained by the RSM model. Also, the root mean square error (RMSE) observed by the ANN model was 0.0099 while the RMSE observed by the RSM model was 0.1871. Since a lesser RMSE shows the greater efficiency of a model in predicting the corrosion rate of mild steel occurring in crude oil solutions. Therefore, based on the R2 and RMSE values observed in this study with varying concentrations of the binary inhibitor, time and pH, ANN demonstrated a better efficiency than the RSM model in predicting the corrosion rate of mild steel in crude oil.
Furthermore, both the models (RSM and ANN) used in this study are compared in Fig. (6). Fig. (6) depicts the experi-mental and predicted plots for each model. From the figure, it is obvious that the trained ANN model is more effective in predicting the corrosion rate of mild steel in crude oil solutions. The better predictive capacity of the ANN model is as a result of its potential to adequately approximate the system non-linearity, in contrast to the RSM model which is limited to second-degree polynomial [15, 22]. Although, when the ANN technique is applied, it must be observed that its predictions are limited to the range of process factors considered in the training process [23].
The optimum condition of variables established by both models included pH 5, 30 mg/L of the concentration of binary inhibitor and 13 days of time. This essentially resulted in the lowest corrosion rate of mild steel at 0.04 mm/yr. Thus, this validates the efficiency of the binary inhibitor on mild steel in crude oil solutions.
CONCLUSION
In this study, the corrosion efficiency of a binary mixture of sesame and castor oil was considered. The inhibitory effects of the binary inhibitor on mild steel in crude oil solutions were considered using different process parameters, which were the concentration of binary inhibitor, pH and time. The optimum inhibition efficiency of 0.04 mm/yr was established at pH 5, 30 mg/L of the concentration of binary inhibitor and time of 13 days. Results evidenced that the binary inhibitor showed an excellent performance in inhibiting the corrosion rate of mild steel in brine solutions. Comparing the predictive capacity of both the models in this study, ANN demonstrated to be a better predictive optimization tool to determine the corrosion rate of mild steel in crude oil solutions rather than the RSM model.
CONSENT FOR PUBLICATION
Not applicable.
AVAILABILITY OF DATA AND MATERIAL
The authors confirm that the data supporting the findings of this study are available within the article.
FUNDING
None.
CONFLICT OF INTEREST
The authors declare no conflict of interest, financial or otherwise.
ACKNOWLEDGEMENTS
The authors are grateful to the management of Covenant University, Ota, Nigeria for sponsoring the publication of this article.